Monetary Policy Based on Biased Evidence
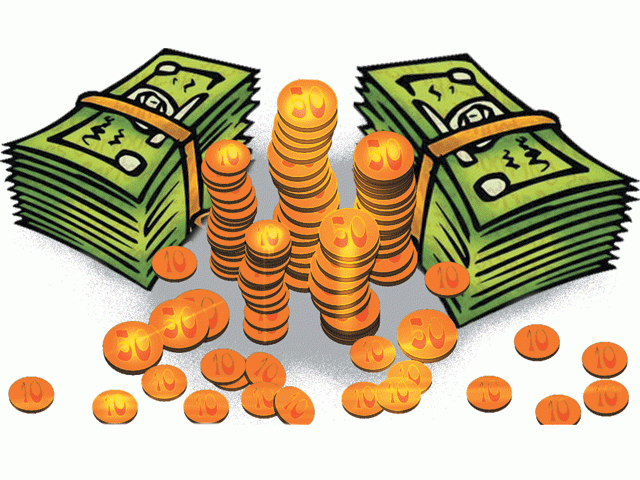
Introduction
A central social planner makes economic decisions which affect the people’s well-being. These decisions determine resource allocation, income distribution and other issues relevant to people’s everyday lives. One such robustly centralised social planner, having a great deal of influence, is a central bank (in the case of Georgia, it would be the National Bank) since the decisions it makes affect the society at large and determine the fates of many.
Almost everywhere, a central bank is a bureaucratic body equipped with massive powers. Most importantly, a central bank has delegated the authority to issue money and monopoly on legal tender. This is precisely how the latter affects resource allocation and income distribution in the economy. In addition, as a lender of last resort, it also determines potential winners and losers in the economy. By employing various monetary instruments, a central bank also influences the issuance and distribution of loans. It is also in charge of regulating the activities of commercial banks. It, therefore, is authorised to impose sanctions on financial institutions, demand changes in the executive body, replace management with an administrator appointed by its own or revoke the license of any commercial bank altogether. This a body exercising complete control over the financial sector, usually under collegial governance.
The Decision-making process in the National Bank of Georgia (NBG) is different compared to other central banks. In the case of the NBG, important decisions are made personally by the Governor of the NBG. At the same time, the role of its supreme body – board and board members – is minimal in implementing monetary and prudential policies. In short, NBG is a non-democratic, bureaucratic-technocratic administrative entity vested with a broad mandate of powers.
The following parts will offer an overview of the economic models and their role in decision-making, testing economic theories and assumptions as well as bias in existing economic literature, based on which a central bank makes a decision or justifies others which have already been made.
Social Planner
A social planner, through the lens of welfare economics, is a decision-maker who tries to achieve the best possible outcome of a decision for everyone. Any “benevolent” social planner aims to maximise social benefits. Ideally, benefit maximises when one individual’s situation improves, at least without the expense of worsened condition of the other. However, in the case of a central bank, this a-prior cannot be achieved because, in the modern (fractional reserve) banking system, the outcome of a monetary policy changes the general price level in the economy. The result of price level changes is an arbitrary and random distribution of incomes (Romer & Romer, 1998; Dolmas et al., 2000; Bagchi et al., 2019; Mumtaz & Theophilopoulou, 2020) when improvement of one’s situation worsens the situation of the other. However, it is also unlikely, even theoretically, that the marginal profit of “winners” in this distribution exceeds the marginal loss of “losers”. For instance, other things being equal, if a central bank increases the money supply, it will cause inflation, that is, price level growth. Inflation is such tax when the relatively poor majority is being levied to subsidise wealthier minorities.
Nevertheless, a social planner needs to be equipped with relevant information and knowledge to ensure the amplification of the welfare of society. Therefore, a central bank should bear similar characteristics. However, banks are also devoid of such knowledge with other central planners. For instance, monetary decisions constitute a policy of the demand side. As clarified by several central bankers, monetary policy is active when changes in the general price level in the economy are affected by demand factors and remains passive when it is a result of a supply shock. The arguments of some of the New Keynesian authors also tend to indicate that (Aoki, 2001; Benigno, 2004; Blanchard & Galí, 2007). It is believed that if a supply shock causes price level growth in the economy, then monetary policy intervention will bring losses in social welfare will be higher than benefits. However, it requires measuring and comparing losses and benefits before and after the implausible intervention.
Central banks guide themselves with economic models that contain variables lacking the possibility of measurement. These variables are hypothetically divided into the supply side and demand side. The monetary policy responds to the latter, tackling a task that is impossible to accomplish.
Since it is a very abstract notion, it is impossible to measure demand, let alone aggregate demand which is a central variable of the macroeconomic models. Demand is defined as a wish and readiness of a consumer to purchase a certain amount of goods and services at a given price. As it is impossible to measure the former, instead of aggregate demand, economists and mathematicians use private consumption, investments, government expenses and net exports for empirical testing and policy analysis of the macroeconomic model. For a shorter period, aggregate output is commonly used. Henceforth, the decisions of monetary authorities are based on such macroeconomic models.
It is vital to have an extensive discussion about those economic models and their validity which are used to justify decisions made in the field of public administration. Their analysis helps to illustrate, based on what standards and knowledge a social planner – in this case, a central bank – makes a decision which is reflected on every member of society, affecting their incomes and distribution of the latter.
Economic Models
Economists draw models for studying the economic phenomenon, exhibiting the process and forecasting the outcomes of the decisions. They mostly use mathematical languages and graphic illustrations to show these models. The models themselves are based on several assumptions. These assumptions are precisely what constitutes a significant problem of the economic models. Economic schools and some economists debate about the plausibility of assumptions. For instance, decision-making among humans assumes to be rational. However, followers of behavioural economics oppose such a stance and claim that people sometimes also make irrational decisions.
The macroeconomic models are also based on some assumptions. For instance, most monetary policy models stem from an assumption that prices are rigid. However, some economists argue that prices are not characterised by rigidity. For some, it is a level of rigidity that differs; for others, it is a period of rigidity, etc (Laidler, 1996). One group of public choice models subscribe to an assumption that government is “benevolent” and its intervention and policy are inexorably congruent with the preferences of a population. On the contrary, others argue that political power is the “leviathan” (Brennan & Buchanan, 1977; 1980). However, the government is not a “benevolent” but a monopolistic entity focused on maximising income. The latter is achieved at the expense of excessive taxation of individuals.
From this standpoint, economic models that tend to favour the government’s active intervention enjoy support from political authorities. Therefore, such models become dominant owing to the government’s direct or indirect efforts, often without empirical support. These models are then used to make ex-ante decisions or justify ex-post decisions. Thus, it is essential to conduct empirical testing of some assumptions of the economic theories and models and obtain evidence to either validate or negate them.
The Role of Econometrics
Scholarly articles of the last decades are primarily devoted to finding empirical evidence with math as a language of communication. As mentioned earlier, economists use math as a tool of abstraction to describe and present their models. Therefore, the economic models are written down in a math language, whereas model assumptions are presented similarly. This is where math and statistics penetrate through economics, and this is where econometrics comes into play. The function of econometrics, first and foremost, is to formulate the economic model to make its empirical testing possible. For instance, the production model is presented as a function of labour, and capital y=f.(L,K). Theoretically it is accurate but empirical testing and future application of that is impossible. The reason is the issue of measurement of variables used in the model. For instance, in what units should capital and the problem of its heterogeneity be measured? This problem is further aggravated when it concerns the macro level, and its practical application begins.
The econometric formulation of the abovementioned mode of production could be as follows: y=a+bL+bK1+bK2+bK3+ … bKn+e. However, this form's equation looks unrealistic because if labour and capital are zero, it is physically impossible to have output. The second easily discernible problem is the variable of capital which requires measurement and solution of heterogeneity problem, which is impossible in practice. Since the measurement of variables in several economic models is impossible, they are substituted with other measurable intermediate variables. This further detaches a specific economic model from the real-world and aggravates the problem. Intermediate variables are amply used in monetary policy models (Rudd & Whelan, 2007; Stock & Watson, 2008; Abbas et al., 2016) and central bank’s prospective use assessors. For instance, to forecast mid-term inflation, central banks often use a novel hybrid Philips Curve-type equation which includes supply shock. Changes in oil prices and food are used as an intermediate variable for the latter. This itself is a part of changes in the general price level. It is the model that the NBG uses for forecasts and making monetary decisions based on those forecasts (Tvalodze et al., 2016).
Publication Bias
On top of the abovementioned problematic issues, when it comes to testing the validity of the macroeconomic models as well as policy based on those models, there is a widespread and sharply manifested cases of publication bias in the empirical literature (Brodeur et al., 2016; Ioannidis et al., 2017; Blanco-Perez & Brodeur, 2020). There can be plenty of reasons behind publication bias, which is hypothetically divided into two types and concerns about publication bias come from an early period (Tullock, 1959).
Type I bias includes primary measurement errors, missing variables, selection of econometric specificity of the model and other similar issues. Type I bias results in different works having different assessments of the same economic phenomenon. Association/effect assessments between the variables may differ in character, size, or statistical significance. This can be caused by different variables measurements, using different proxy variables, selecting different regressors, etc. For instance, empirically calculated parameters of monetary policy models will differ on which variable will be used as a proxy for aggregate demand.
In addition, missing variable problems can also be a reason for different results in the empirical literature on the same phenomenon. This problem persists when a vital variable is missing from an econometric model. However, another widespread occurrence is when the same variables have varying interrelationships, or there is a sharp difference in the level of relationship between them. The reason for that is the specificity of an assessor model. Using the same variables in models of different specificity produces different outcomes. The authors themselves select these models and seek to explain their validity of the models. Therefore, the author’s selection of an assessor of relationships between the model variables is inclined to obtain empirical support for the position preferable to the author.
Type II publication bias is about the statistical significance of the authors’ findings and their conformity with a dominant economic theory. Suppose empirical study results of an economic phenomenon are statistically insignificant and incongruent with a dominant theory. In that case, a “file drawer” problem may arise (Rose & Stanley, 2005), resulting from the author’s, the journal’s editor’s or referee’s action. On the one hand, editors and referees may tend to accept articles in line with conventional views. On the other hand, authors may use traditionally anticipated results as a validity test for an econometric model and assessor they selected. Finally, everyone, including authors, editors and referees, can have attitudes of favouritism to “statistically significant” results. For the latter, authors may resort to “specification search”. Leamer (1978) highlights authors’ inclination to “specification search” in non-experimental research studies.
The dominance of any economic theory complicates the substantiation of empirical findings that contradict the hegemonic position. Therefore, authors are less inclined to present results which sharply contradict widely accepted theories. In other words, when there is a monopoly over a specific economic model, authors have to pay higher prices to present more robust, more dramatic, statistically significant results. Doucouliagos and Stanley (2011) studied this issue and demonstrated a direct relationship between the disputability of an economic theory and selective bias. In particular, based on an analysis of empirical literature on 87 separate fields in the economy, they concluded that in economic theory, there is a negative relationship between competition and publication bias. Analysis of authors indicates that the more dominant a position vis-à-vis a specific economic phenomenon is more biased empirical literature is in this respect. Among the areas mentioned are the so-called Taylor's rule - inflation and release mechanism, which according to the authors' classification, is non-controversial, non-competitive, and at the same time, it is empirically shown, is characterised by the highest bias. The inflation mark is especially noticeable.
NBG also subscribes to Taylor’s principle, which suggests implementing a rules-based monetary policy. The NBG's forecasting and monetary policy analysis system indicates that policy is based on Taylor-type rules (Tvalodze et al., 2016).
Career Incentive
For the last decade, there has been an exponential rise in the studies of publication bias in the empirical literature as well as assessments of relationships between variables beyond bias via correcting the abovementioned (Havránek et al., 2020). The works devoted to this issue also include a search for global “reasons” behind the bias. Paldam (2018) argues that economists act like politicians and bureaucrats. Public choice economists believe that politicians and bureaucrats work not only to maximise social benefit but also to have parochial other interests. This is the same case for economists too, whose aim is not a search for truth alone but they are animated by other interests as well. These interests may lead them to exaggerate the results of empirical work. Frey (2003) notes that success in the academic field and continued presence depend on publishing articles in some of the most-cited journals. In contrast, publication depends on the satisfaction of the demands of some unknown referees. Therefore, authors’ career incentives may be a reason behind publication bias.
Publication bias studies demonstrated that career aims could be ground for exaggeration of effect and inclination to show statistically significant results. Doucouliagos et al. (2022), through a meta-analysis of the empirical literature on aid effectiveness, demonstrated that career incentive affects publication decisions. Elderly and well-respected authors tend to publish less biased assessments in the literature on aid effectiveness than others.
Assessment of the central banks’ policy impact is not an exception regarding bias. The empirical literature on monetary policy also tends to have a publication bias. Several meta-analyses show that the effect of a conventional monetary policy (price growth and output) is primarily biased in favour of a dominant position (Rusnák et al., 2013; Nguyen, 2019; Nguyen et al., 2021). Here, of interest, is the assessment trends of non-conventional monetary policy pursued by the central banks in the wake of the 2008-2009 world financial crisis.
In the aftermath of the 2008-2009 crisis, the central banks started to implement active non-conventional policy, followed by empirical study in the subsequent years. The primary instrument of such a policy is quantitative easing. Fabio et al. (2021) studied empirical literature on quantitative easing. Based on the analysis of the results of 54 works, these authors concluded that the central banks’ works show quantitative easing to be more effective than academic publications. In particular, the central banks’ publications show a more significant impact of quantitative easing on inflation and output than the others. Their research also reflects on the statistically significant effect on output. A non-conventional policy aimed to facilitate output growth in the post-crisis period. One more exciting and substantial finding is that the central banks’ employees, who succeed in demonstrating a higher effect of quantitative easing on output, enjoy better career prospects (Fabo et al., 2021).
Publication bias has been detected not only in monetary policy but also in the bank supervision component. Film and Lind (2020) analysed empirical literature on Basel III’s macroeconomic impact. In particular, a meta-analysis of 48 works on macroeconomic price for higher capital (buffer) requirements for commercial banks identified that empirical literature is afflicted with a publication bias. Authors believe that the strong consensus that tighter capital requirements on banks will negatively affect a real economy could be the reason. According to the authors, another important finding of that work is the distribution of average indicators of results in the empirical literature. For instance, authors affiliated with financial system regulatory bodies (-0.060), International Monetary Fund (-0.141) and Central Bank (-0.175) say that tightening minimal capital requirement will have a little negative impact on the economy, whereas authors who are connected with the banking sector indicate on the contrary that this measure will have a high adverse effect (-0.425).
Concluding Remarks
Monetary decisions of a central bank, as a social planner, fails to meet even reasonable suspicion standard because it does not have the necessary evidence for that. Furthermore, on the one hand, economic models used by the central banks remain distanced from the real world. On the other hand, empirical testing literature that serves as a theoretical basis for the central banks’ policies suffers from a publication bias. Career goals are often the reason behind publication bias.
In these circumstances, the central banks make monetary policy decisions with far-reaching consequences for society. This is a political and legal problem. This massive power of banks is justified by the need to implement knowledge-based policy by technocratic-bureaucratic administration. However, the latter suffers precisely from the lack of relevant knowledge and information. On the other hand, decisions made are based on evidence characterised by several biases.
Therefore, it is unjustified to delegate broad monetary authority to a central bank, as a non-elected technocratic-bureaucratic administration lacks democratic legitimacy because it does not have direct political accountability and is not representative. This problem is even more severe in Georgia. As opposed to other central banks, the monetary policy of the NBG is not a matter of collegial decision-making (which would have higher legitimacy since the supreme body – either board or committee – is staffed with members nominated by the President and elected by the Parliament), because the whole monetary authority is delegated personally to the President of the NBG.
References:
Abbas, S. K., Bhattacharya, P. S. and Sgro, P. (2016). The new Keynesian Phillips curve: An update on recent empirical advances. International Review of Economics &Amp; Finance, 43, 378–403. https://doi.org/10.1016/j.iref.2016.01.003
Aoki, K. (2001). Optimal monetary policy responses to relative-price changes. Journal of Monetary Economics, 48(1), 55–80. https://doi.org/10.1016/s0304-3932(01)00069-1
Bagchi, S., Curran, M. and Fagerstrom, M. J. (2019, September). Monetary growth and wealth inequality. Economics Letters, 182, 23–25. https://doi.org/10.1016/j.econlet.2019.05.036
Benigno, P. (2004). Optimal monetary policy in a currency area. Journal of International Economics, 63(2), 293–320. https://doi.org/10.1016/s0022-1996(03)00055-2
Bernanke, B. S. (2020). The New Tools of Monetary Policy. American Economic Review, 110(4), 943–983. https://doi.org/10.1257/aer.110.4.943
Blanchard, O. and Galí, J. (2007). Real Wage Rigidities and the New Keynesian Model. Journal of Money, Credit and Banking, 39, 35–65. https://doi.org/10.1111/j.1538-4616.2007.00015.x
Blanco-Perez, C. and Brodeur, A. (2020). Publication Bias and Editorial Statement on Negative Findings. The Economic Journal, 130(629), 1226–1247. https://doi.org/10.1093/ej/ueaa011
Brennan, G. and Buchanan, J. M. (1977). Towards a tax constitution for Leviathan. Journal of Public Economics, 8(3), 255-273. https://doi.org/10.1016/0047-2727(77)90001-9
Brennan, G. and Buchanan, J. M. (1980). The power to tax: Analytic foundations of a fiscal constitution. Cambridge University Press.
Brodeur, A., Lé, M., Sangnier, M. and Zylberberg, Y. (2016). Star Wars: The Empirics Strike Back. American Economic Journal: Applied Economics, 8(1), 1–32. https://doi.org/10.1257/app.20150044
Dolmas, J., Huffman, G. W. and Wynne, M. A. (2000). Inequality, inflation, and central bank independence. Canadian Journal of Economics/Revue Canadienne D`Economique, 33(1), 271–287. https://doi.org/10.1111/0008-4085.00015
Doucouliagos, C. and Stanley, T. (2011). ARE ALL ECONOMIC FACTS GREATLY EXAGGERATED? THEORY COMPETITION AND SELECTIVITY. Journal of Economic Surveys, 27(2), 316–339. https://doi.org/10.1111/j.1467-6419.2011.00706.x
Doucouliagos, H., Hinz, T. and Zigova, K. (2022). Bias and careers: Evidence from the aid effectiveness literature. European Journal of Political Economy, 71, 102056. https://doi.org/10.1016/j.ejpoleco.2021.102056
Fidrmuc, J. and Lind, R. (2020). Macroeconomic impact of Basel III: Evidence from a meta-analysis. Journal of Banking &Amp; Finance, 112, 105359. https://doi.org/10.1016/j.jbankfin.2018.05.017
Frey, B. S. (2003). Publishing as prostitution?–Choosing between one's own ideas and academic success. Public choice, 116(1), 205-223. https://doi.org/10.1023/A:1024208701874
Havránek, T., Stanley, T. D., Doucouliagos, H., Bom, P., Geyer‐Klingeberg, J., Iwasaki, I., Reed, W. R., Rost, K. and Aert, R. C. M. (2020). REPORTING GUIDELINES FOR META‐ANALYSIS IN ECONOMICS. Journal of Economic Surveys, 34(3), 469–475. https://doi.org/10.1111/joes.12363
Ioannidis, J. P. A., Stanley, T. D. and Doucouliagos, H. (2017). The Power of Bias in Economics Research. The Economic Journal, 127(605), F236–F265. https://doi.org/10.1111/ecoj.12461
Laidler, D. (1996). Wage and Price Stickiness in Macroeconomics: Historical Perspective. Monetary Economics in the 1990s, 92–121. https://doi.org/10.1007/978-1-349-25204-6_6
Leamer, E. E. (1978). Specification Searches: Ad Hoc Inference with Nonexperimental Data (1st ed.). Wiley.
Martin, C. and Milas, C. (2012). Quantitative easing: a sceptical survey. Oxford Review of Economic Policy, 28(4), 750–764. https://doi.org/10.1093/oxrep/grs029
Mumtaz, H. and Theophilopoulou, A. (2020). Monetary policy and wealth inequality over the great recession in the UK. An empirical analysis. European Economic Review, 130, 103598. https://doi.org/10.1016/j.euroecorev.2020.103598
Nguyen, T. M. L. (2019). Output Effects of Monetary Policy in Emerging and Developing Countries: Evidence from a Meta-Analysis. Emerging Markets Finance and Trade, 56(1), 68–85. https://doi.org/10.1080/1540496x.2019.1601081
Nguyen, T. M. L., Papyrakis, E. and van Bergeijk, P. A. (2021). Publication bias in the price effects of monetary policy: A meta-regression analysis for emerging and developing economies. International Review of Economics &Amp; Finance, 71, 567–583. https://doi.org/10.1016/j.iref.2020.09.010
Paldam, M. (2018). A model of the representative economist, as researcher and policy advisor. European Journal of Political Economy, 54, 5–15. https://doi.org/10.1016/j.ejpoleco.2018.03.005
Romer, C. and Romer, D. (1998). Monetary Policy and the Well-Being of the Poor. NBER Working Paper. https://doi.org/10.3386/w6793
Rose, A. K. and Stanley, T. D. (2005). A Meta-Analysis of the Effect of Common Currencies on International Trade*. Journal of Economic Surveys, 19(3), 347–365. https://doi.org/10.1111/j.0950-0804.2005.00251.x
Rudd, J. and Whelan, K. (2007). Modeling inflation dynamics: A critical review of recent research. Journal of Money, Credit and Banking, 39, 155-170. https://doi.org/10.1111/j.1538-4616.2007.00019.x
Rusnák, M., Havranek, T. and Horváth, R. (2013). How to solve the price puzzle? A meta‐analysis. Journal of Money, Credit and Banking, 45(1), 37-70. https://doi.org/10.1111/j.1538-4616.2012.00561.x
Stock, J. H. and Watson, M. W. (2008). Phillips curve inflation forecasts.
Taylor, J. B. (1993). Discretion versus policy rules in practice. Carnegie-Rochester Conference Series on Public Policy, 39, 195–214. https://doi.org/10.1016/0167-2231(93)90009-l
Tullock, G. (1959). Publication Decisions and Tests of Significance—A Comment. Journal of the American Statistical Association, 54(287), 593–593. https://doi.org/10.1080/01621459.1959.10501522
Tvalodze, S., Mkhatrishvili, S., Mdivnishvili, T., Tutberidze, D. and Zedgenidze, Z. (2016). The National Bank of Georgia's Forecasting and Policy Analysis System (No. 01/2016). National Bank of Georgia.
See the attached file for the entire document with relevant links and explanations.